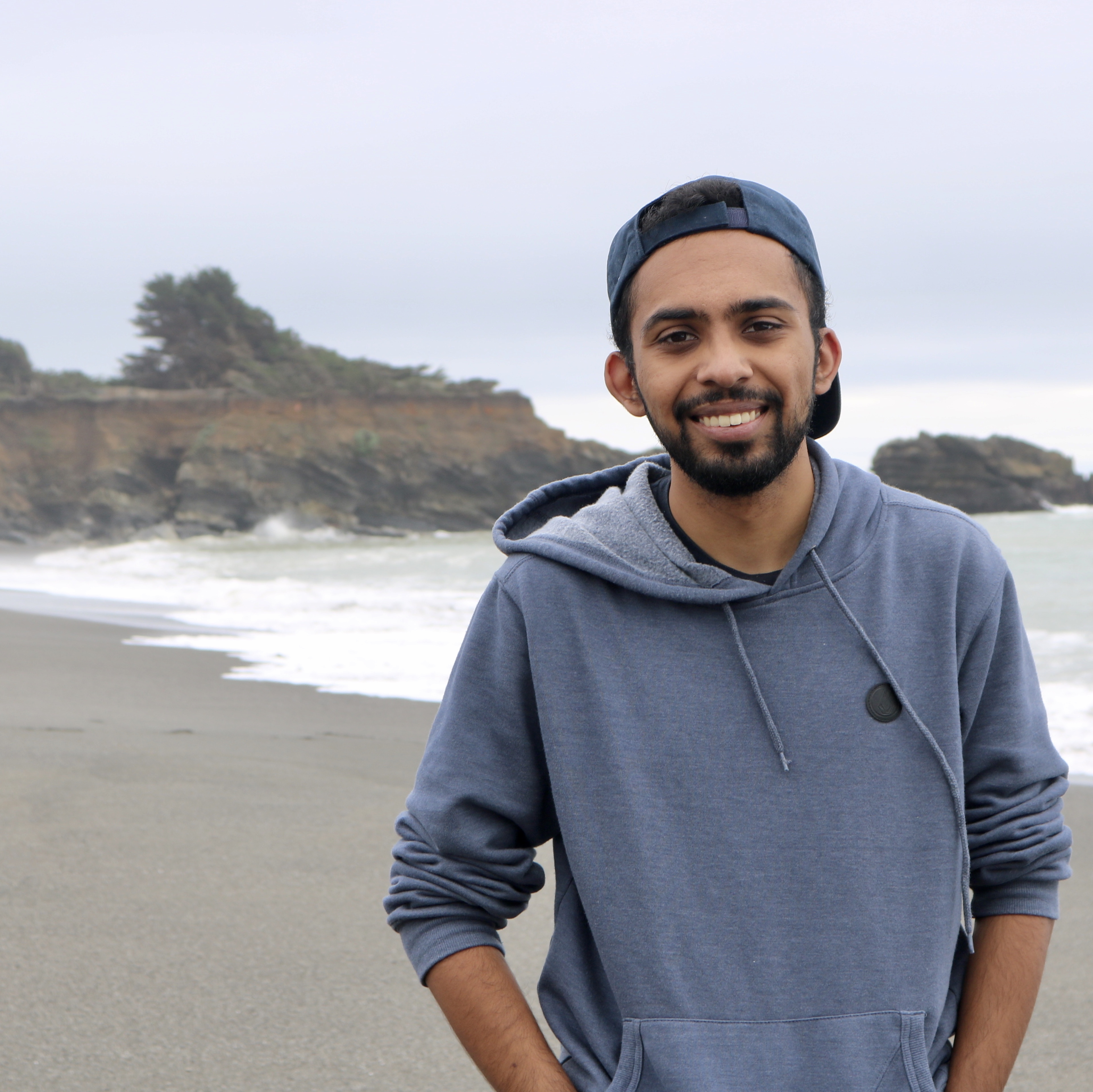
Hi there! I’m Gokul, a PhD candidate in the Robotics Institute at Carnegie Mellon University working on interactive algorithms for agentic alignment (e.g. imitation learning / RLHF).
Rather than continuing to build agents that can only learn when humans tell them the answer, I want to build agents capable of reasoning towards their own answers. In other words, rather than giving agents the “fish”, my research focuses on teaching agents to fish: to make progress towards our desired outcomes even when faced with unforeseen obstacles. I often ground my research in robotics and language modeling.
I work with Drew Bagnell and Steven Wu. I completed my B.S. / M.S. at UC Berkeley, where I worked with Anca Dragan on Learning with Humans in the Loop. I’ve spent summers working on ML @ SpaceX, Autonomous Vehicles @ NVIDIA, Motion Planning @ Aurora, and Research @ Microsoft and @ Google.
In my free time, I like to consume baked goods, go to concerts / art museums, and run / lift. I’m a huge fan of birds (especially lovebirds), books (especially those by Murakami), and bands (especially Radiohead).
Events & News
🌟 June 2025 🌟 - Two new papers out on learning to search: one that introduces SAILOR: a method that outperforms diffusion policies trained on 10x as much human data on multi-stage visual manipulation tasks, another that allows real robots to avoid complex semantic failures via VLM verifiers (accepted to RSS ‘25).
🌟 March 2025 🌟 - New particularly exciting preprint out on the real value of RL in fine-tuning / RLHF. I gave a talk at Cornell on the paper that might also be of interest.
🌟 November 2024 🌟 - Drew, Steven, and I are co-teaching a course on the algorithmic foundations of interactive learning. If you’d like to understand the fundamental principles beyond imitation (e.g. for robots) and RLHF (e.g. for LLMs), this is the course for you!
Research Highlights
A Smooth Sea Never Made a Skilled 𝚂𝙰𝙸𝙻𝙾𝚁: Robust Imitation via Learning to Search
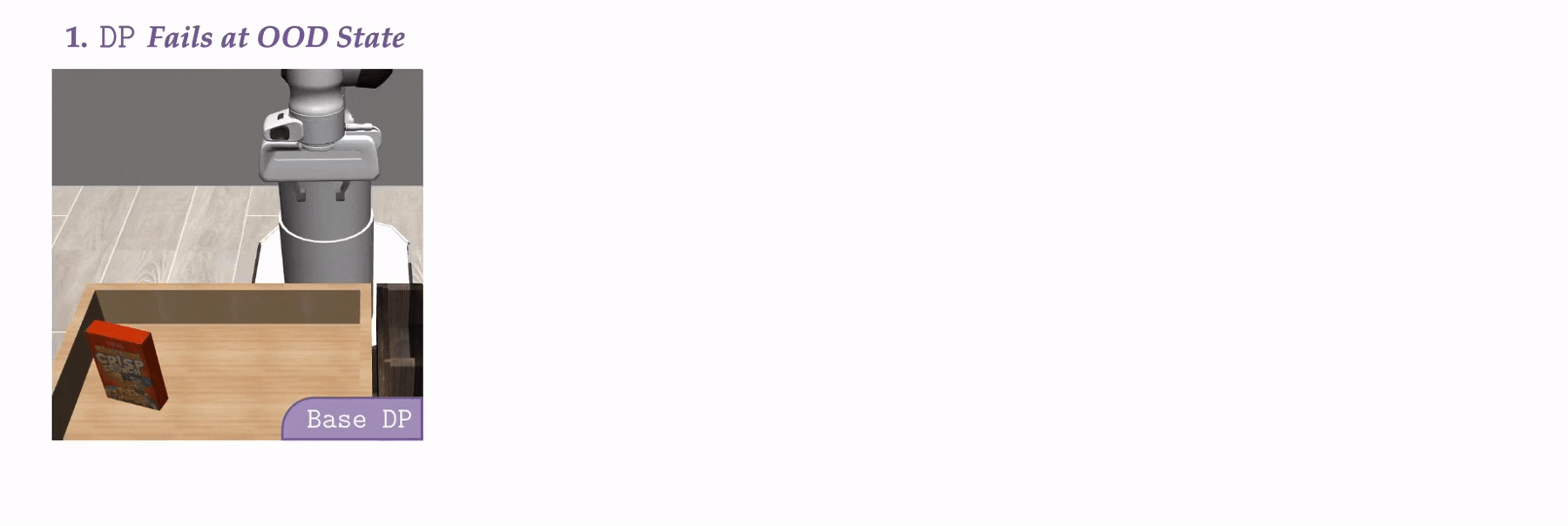
We introduce 𝚂𝙰𝙸𝙻𝙾𝚁: a method for learning to search from expert demonstrations that out-performs Diffusion Policies trained in 5-10x as much data on multi-stage visual manipulation tasks. [Paper] [Site]
All Roads Lead to Likelihood
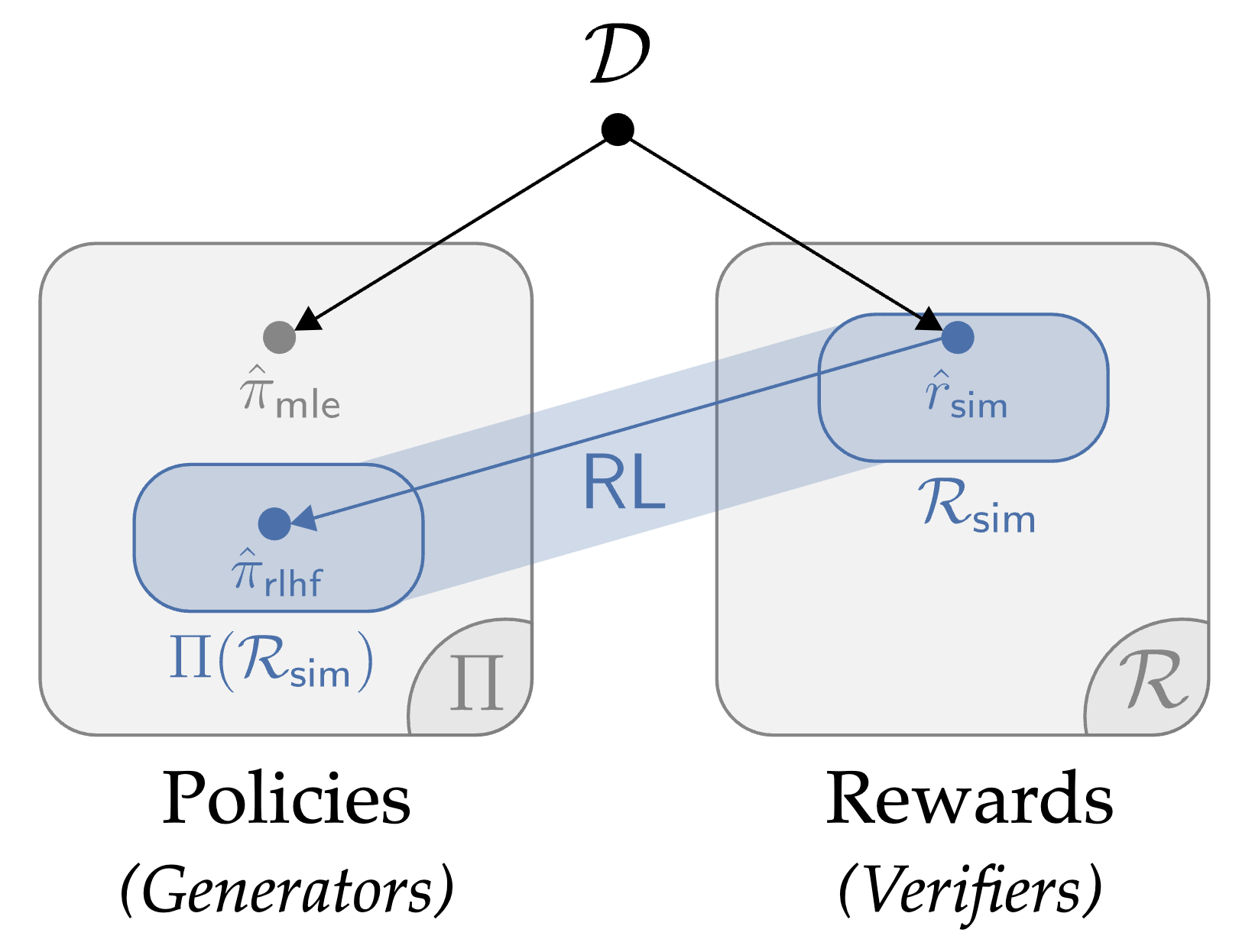
We explore how the value of RL in fine-tuning / RLHF seems to be fundamentally derived from generation-verification gaps. [Paper] [Talk]
SPO: Self-Play Preference Optimization
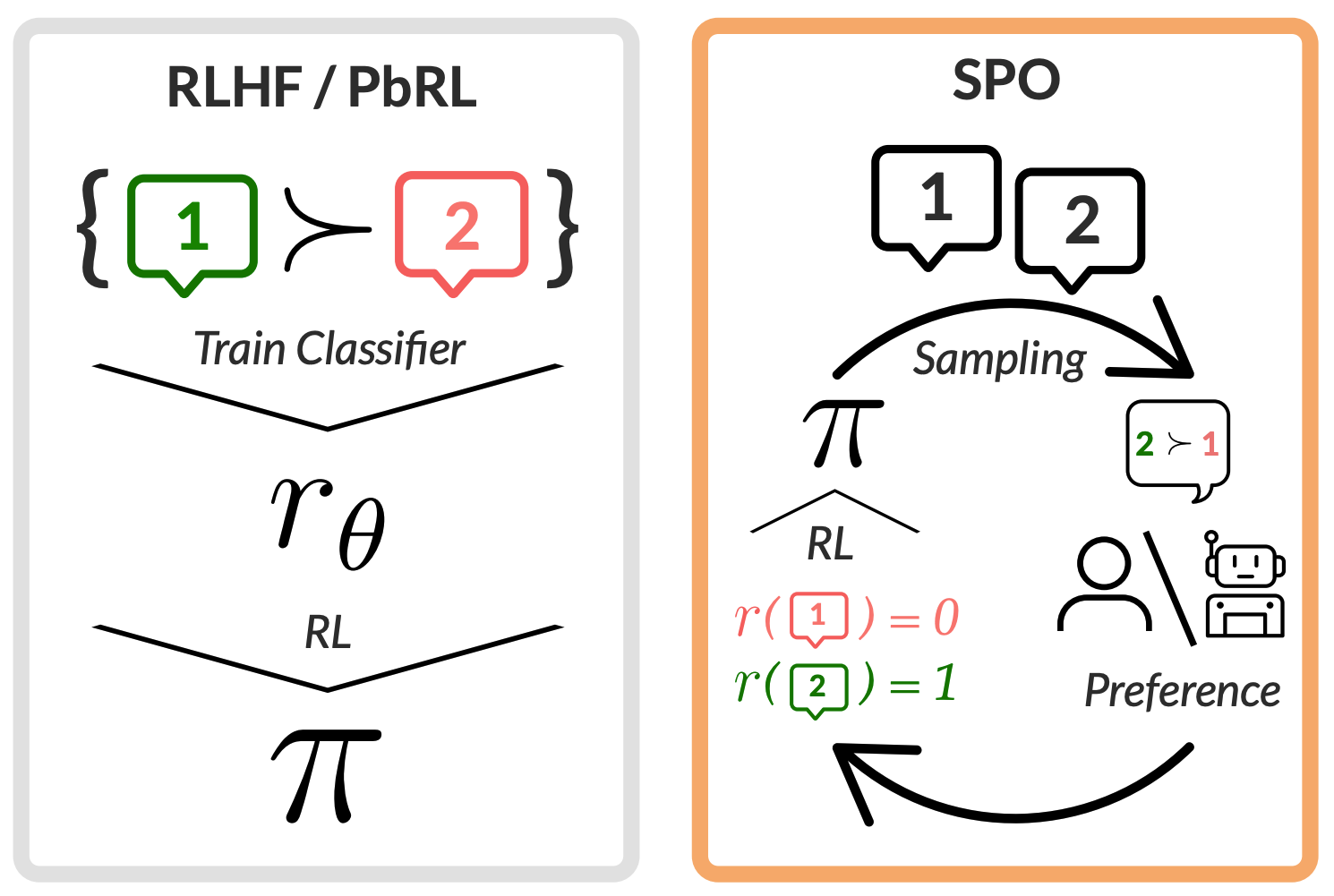
We derive a new fundamental algorithm for RLHF that robustly handles the complex, intransitive preferences that often result from aggregating the a diversity of views. [Website] [Paper][Podcast]
Inverse RL without RL
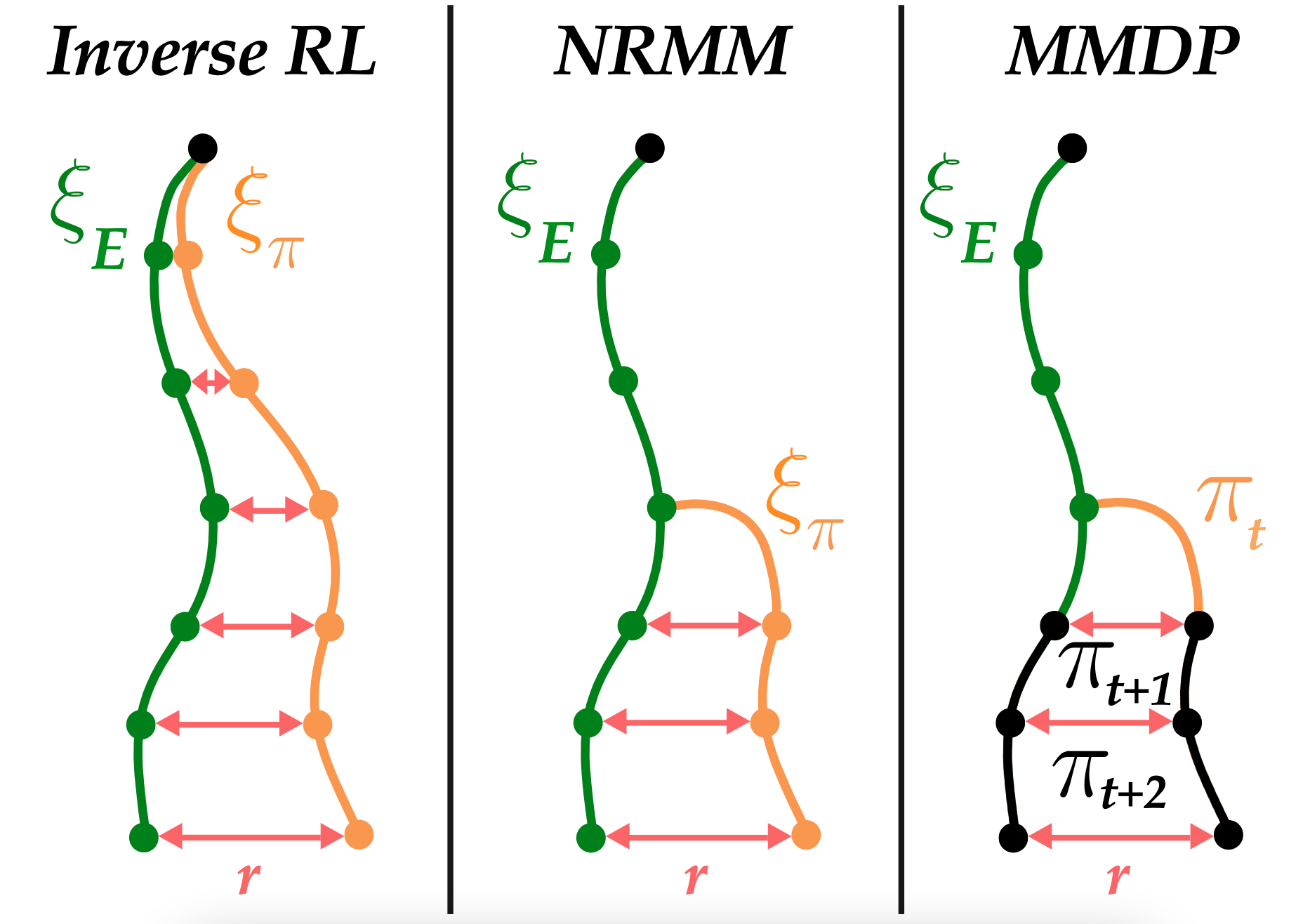
We derive exponentially faster algorithms for inverse RL by proving that local search, rather than global RL, is "all you need" for imitation. [Website][Paper]
Of Moments and Matching
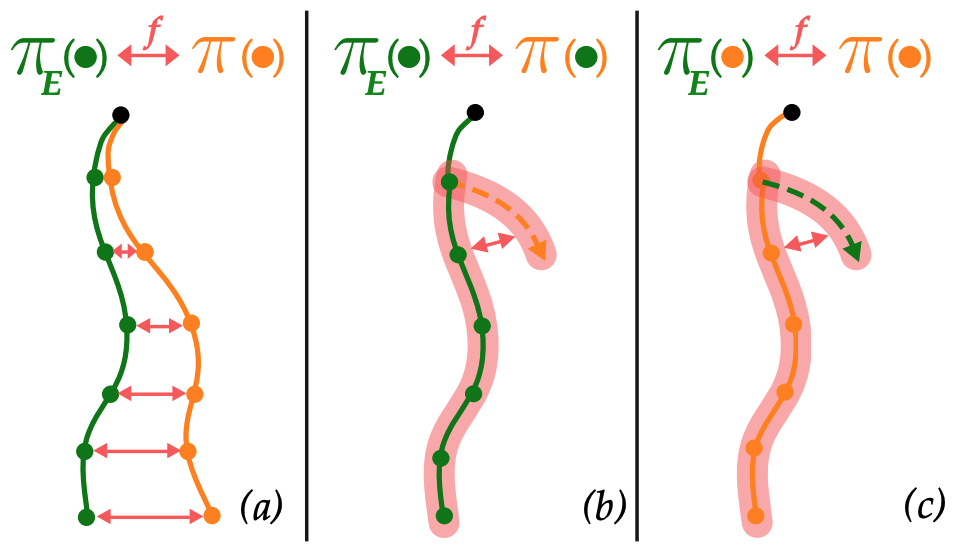
We provide a unifying, game-theoretic framework for imitation learning that explains when different algorithmic families can avoid compounding errors. [Website][Blog]