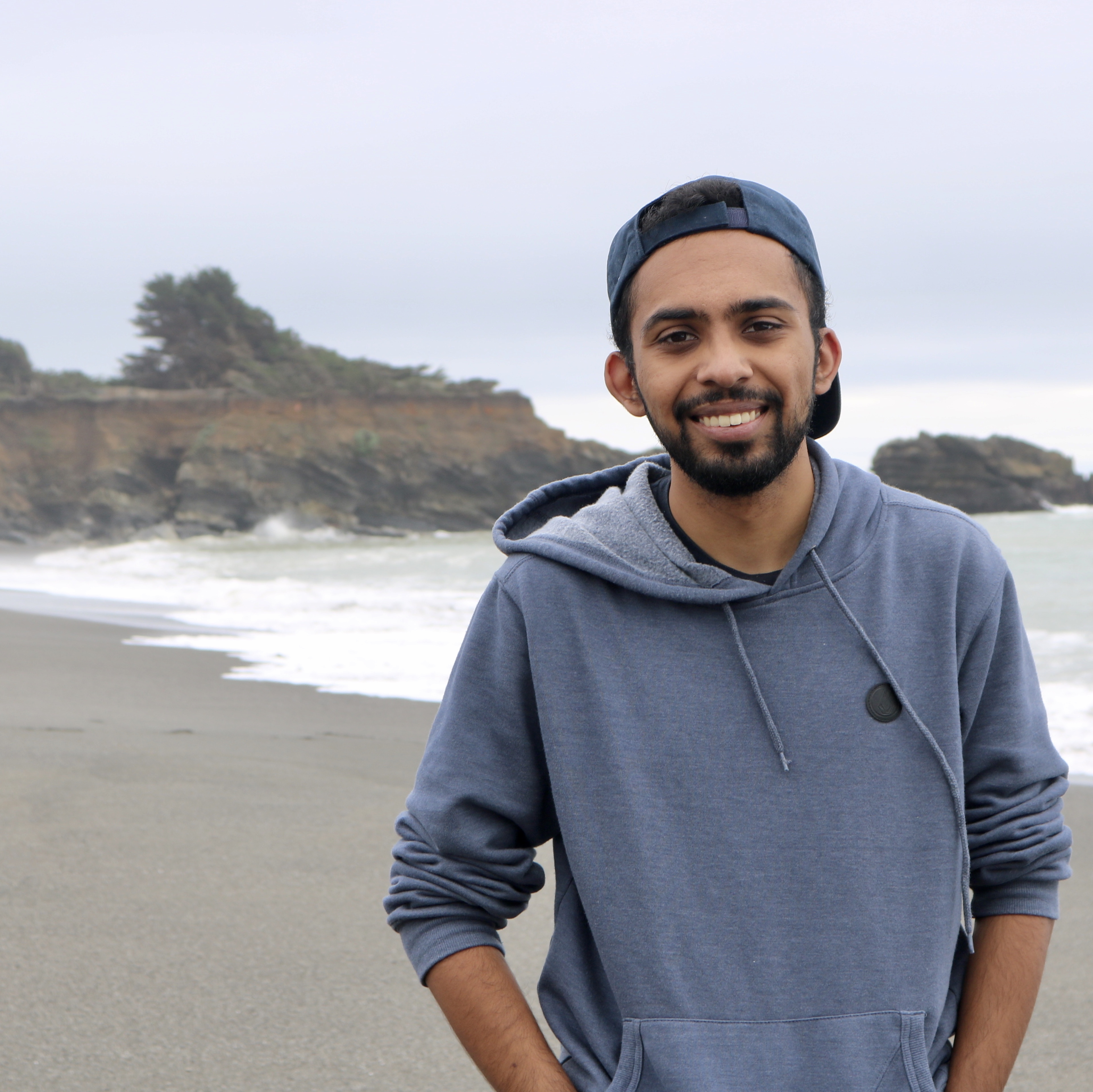
Hi there! I’m Gokul, a PhD candidate in the Robotics Institute at Carnegie Mellon University working on interactive learning from implicit human feedback (e.g. imitation/RLHF).
I work with Drew Bagnell and Steven Wu. I completed my B.S. / M.S. at UC Berkeley, where I worked with Anca Dragan on Learning with Humans in the Loop.
I’ve spent summers working on ML @ SpaceX, Autonomous Vehicles @ NVIDIA, Motion Planning @ Aurora, and Research @ Microsoft and @ Google.
In my free time, I do origami, hackathons, and run/lift. I’m a huge fan of birds (especially lovebirds), books (especially those by Murakami), and bands (especially Radiohead).
If you’d be interested in working with me, feel free to shoot me an email!
Events & News
September 2024 - Three papers accepted to NeurIPS’24! One on what is fundamentally different about multi-agent imitation learning, one on REBEL: a scalable and theoretically elegant RLHF algorithm, and one on the differences between online and offline preference fine-tuning algorithms.
June 2024 - Four papers accepted to ICML’24! One on SPO: an RLHF algorithm for reconciling diverse preferences, one on a fundamental framework for designing efficient inverse RL algorithms, one on evolving reward shaping terms for RL / Inverse RL, and one on transfer learning.
Research Highlights
SPO: Self-Play Preference Optimization
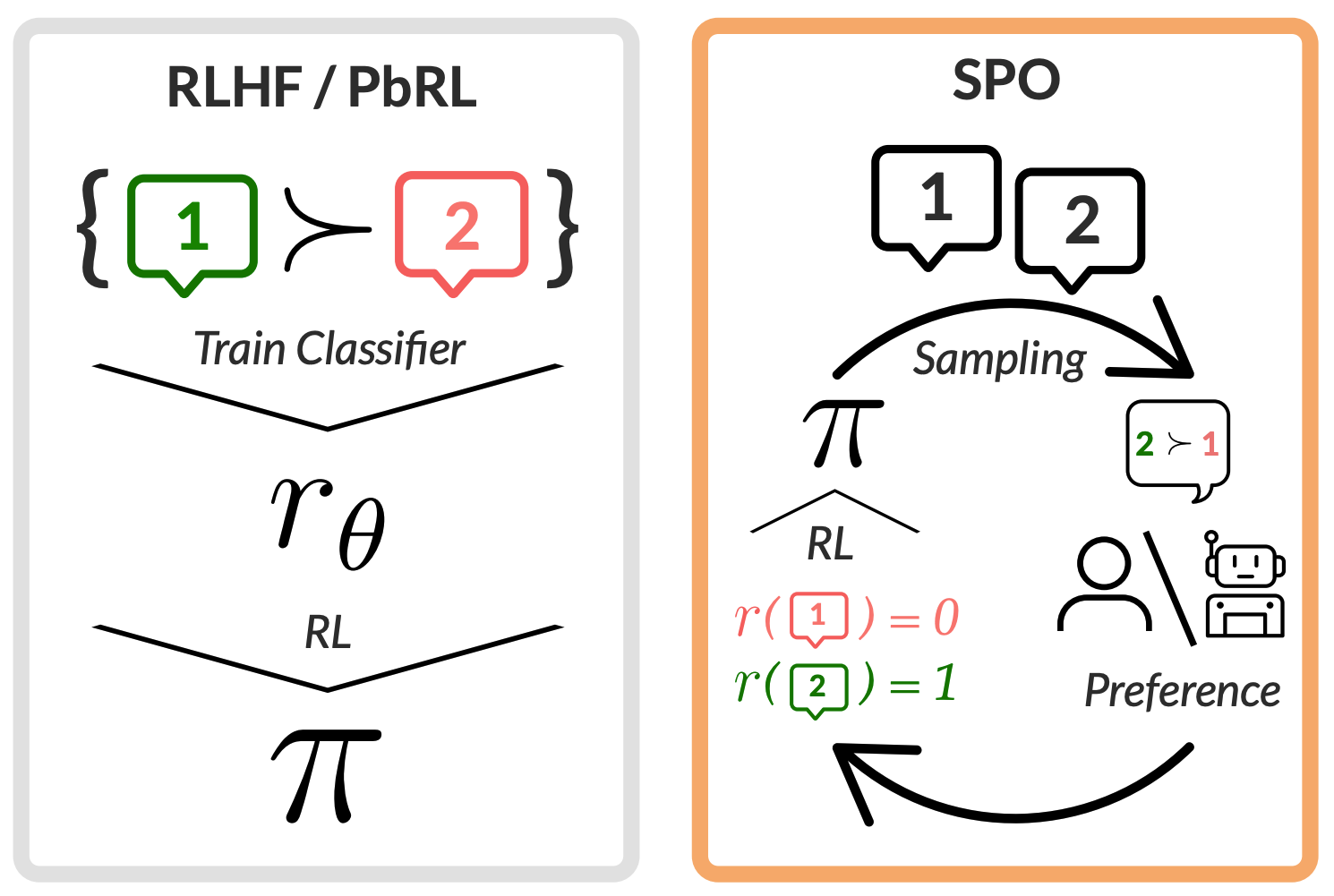
We derive a new fundamental algorithm for RLHF that robustly handles complex intransitive preferences while avoiding reward modeling and adversarial training. [Website] [Paper]
Hybrid Inverse RL
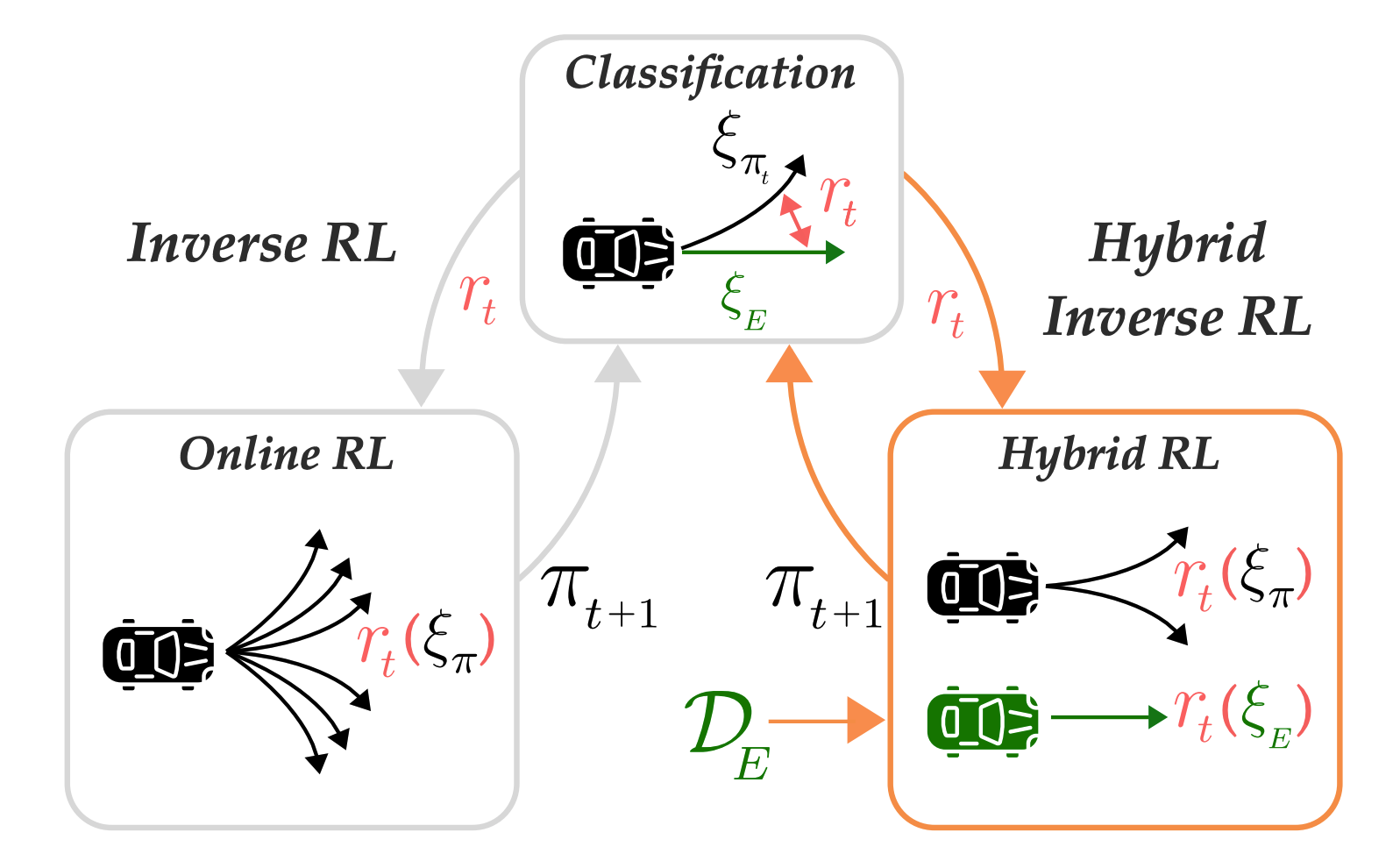
We derive a new flavor of inverse RL that uses expert demonstrations to speed up policy search without requiring the ability to reset the learner to arbitrary states. [Website] [Paper]
Inverse RL w/o RL
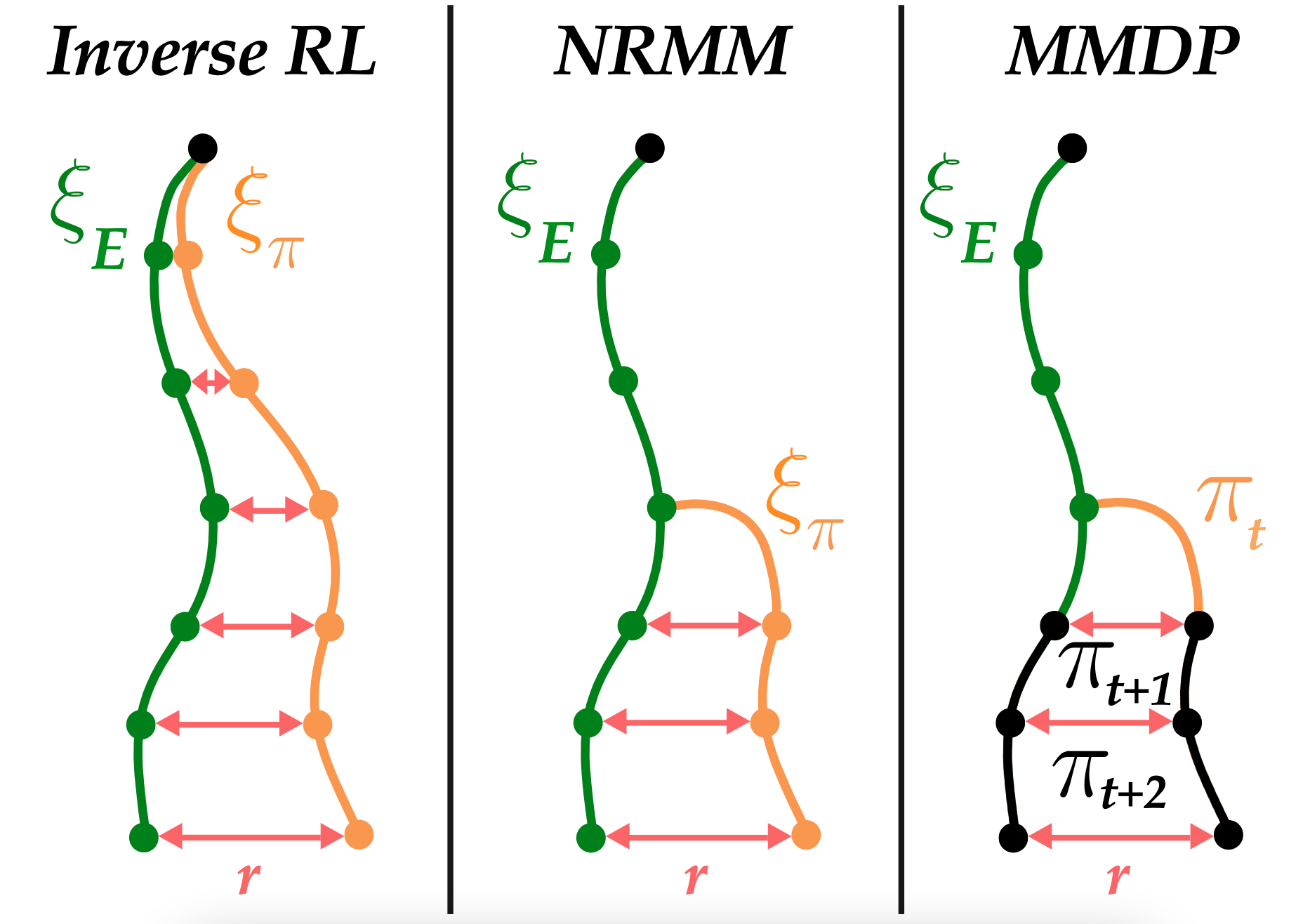
We derive exponentially faster algorithms for inverse RL by resetting the learner to states from the expert demonstrations within the RL subroutine. Our work was published at ICML '23. [Website][Paper]
Imitation w/ Unobserved Contexts
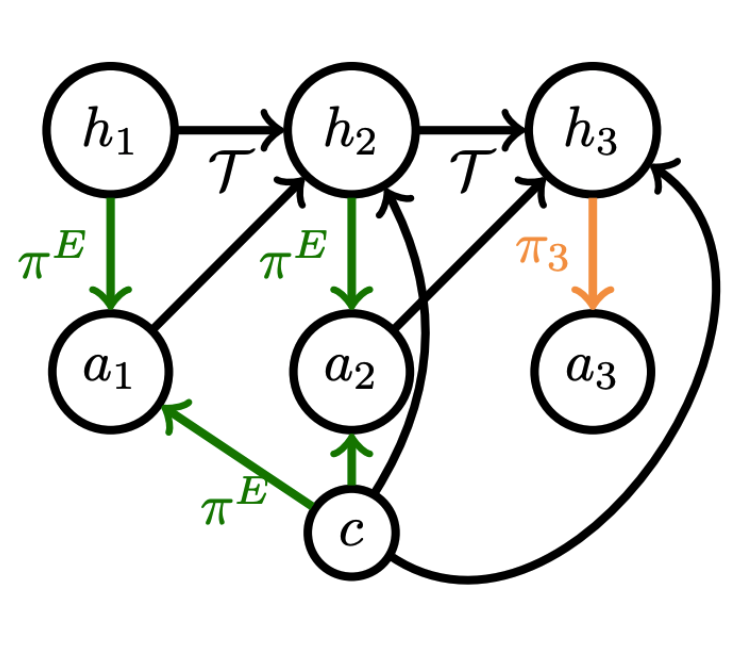
We describe algorithms for and conditions under which it is possible to imitate an expert who has access to privileged information. Our work was published at NeurIPS 2022. [Website][Blog]
Causal Imitation Learning under TCN
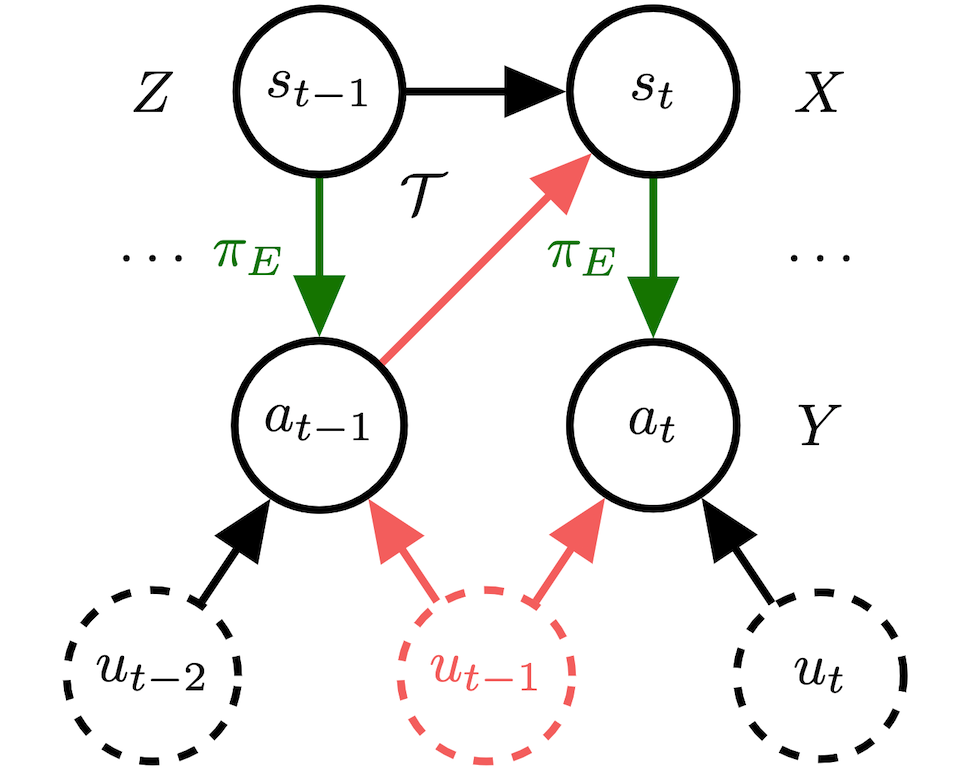
We use instrumental variable regression to derive imitation learning algorithms that are robust against temporally correlated noise both in theory and practice. Oral at ICML 2022. [Website][Paper]
Of Moments and Matching
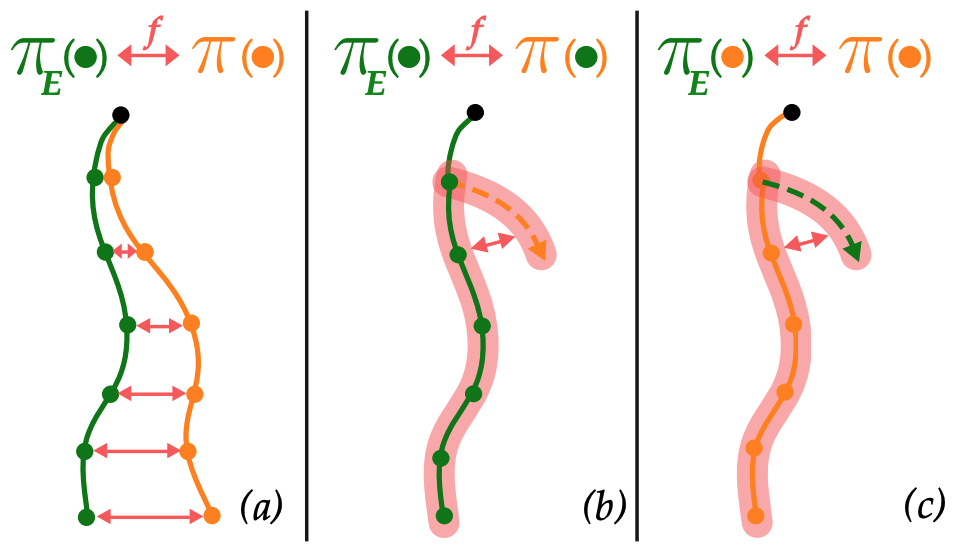
We construct a taxonomy for imitation learning algorithms, derive bounds for each class, construct novel reduction-based algorithmic templates that achieve these bounds, and implement simple elegant realizations with competitive emperical performance. Published at ICML 2021. [Website][Blog]